Beyond FAQ Bots: How AI-Powered Chatbots Are Handling Complex Support Cases
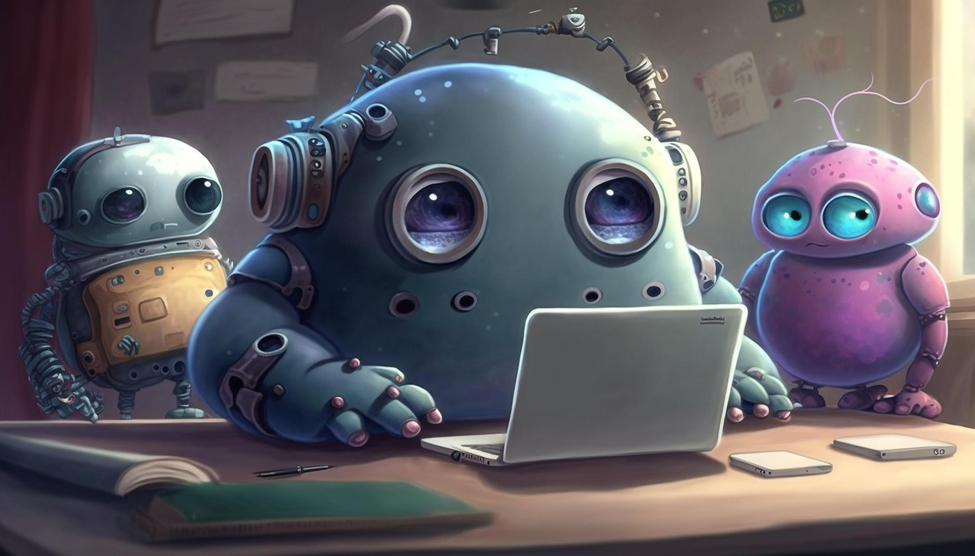
Image by Alexandra Koch at Pixabay
AI customer service solutions are evolving and becoming ever more capable. They’ve gone beyond answering simple questions to providing useful trouble-shooting support.
Do you remember when customer service AI chatbots first came out? They were clunky and relatively inefficient. If you didn’t use the exact right phrase, they didn’t understand what you were talking about. It was also pretty obvious that you were talking to a machine.
Generative AI helped these bots take a giant leap forward. The best AI customer service solutions today can understand the context of an inquiry. These bots can make split-second decisions, and comb through reams of data in seconds. This enables them to manage increasingly complex cases.
Do they have the same problem-solving skills that human consultants do? Not yet, but they’re getting pretty smart. They’re becoming indispensable support tools in customer service by supporting human teams. And, with the help of those teams, they can learn to deal with more complex cases and emulate empathy.
In this post we’ll look at how AI customer service solutions are stepping up to the challenge.
The Evolution of AI-Powered Chatbots
From Rule-Based Scripts to Intelligent Assistants
Early chatbots followed strict rules. If a customer’s question didn’t match a preset script, the bot couldn’t help—leading to plenty of frustration. Today’s AI-driven chatbots are different. They’re smarter, more adaptable, and can handle real conversations. Here’s what makes them stand out:
- Natural language processing (NLP) helps them understand intent rather than just keywords.
- Context retention allows them to remember past interactions.
- Machine learning continuously improves their responses over time.
The Tech Making Bots Even Better
What makes modern AI customer service solutions so efficient?
Natural Language Processing (NLP) and Understanding (NLU)
The earliest form of chatbots worked on simple algorithms. If you used the right keyword, you got the right answer. Advances in NLP make it easier for bots to understand human language more easily. We elevate service levels with NLU, which can:
- Detect emotions like frustration or urgency.
- Extract pertinent details like order numbers, account IDs, or symptoms.
- Clarify vague questions. For example, does, “My account is frozen” mean there’s a problem with the bank account or a problem logging in.
Machine Learning and Reinforcement Learning
Customer service AI chatbots today learn instead of just following scripts. They improve through:
- Supervised learning: You train them on real-world customer interactions. These can be from past support logs, specific datasets, and other similar examples.
- Reinforcement learning: By adjusting their responses based on feedback from your human consultants. For example, AI might suggest two answers to a service agent. The agent will then pick the better one, allowing the AI to learn.
- Self-updating models: These bots adapt to product updates or policy changes.
The combination of the three means that AI never truly stops learning. Which is all for the good.
Multi-Turn Context Retention
Older chatbots had a hard time carrying details forward from previous messages. Transformer models like BERT and GEPT are changing this, allowing bots to keep up with the flow of conversations.
Say, for example, you want to change a flight and ask for new dates. Then you want to know about baggage fees. The chatbot will remember that you’re changing your flight and tailor its response accordingly. This saves you from the frustration of having to repeat yourself.
Integration with Backend Systems
To resolve complex cases, AI customer service solutions must be able to access real-time data. That means connecting with:
- CRM platforms for customer history.
- Order management systems for tracking.
- APIs for instant verification and authentication.
Sentiment Analysis and Emotional Intelligence
The newer bots can read the emotions of a customer. They look for cues that show the client is frustrated and act accordingly. It may adopt a more soothing tone or escalate the matter as quickly as possible.
AI Chatbots in Action: Handling Complex Support Cases
How does this work in the real world?
IT and Software Troubleshooting
A customer reports slow internet. Instead of a generic response, an AI chatbot can:
- Run network diagnostics.
- Check for local outages.
- Walk the customer through troubleshooting steps.
- Escalate to a technician if needed—without making them repeat themselves.
Healthcare and Insurance Assistance
A patient needs to know if their insurance covers a procedure. Instead of digging through paperwork, they:
- Ask the chatbot, which checks their policy in seconds.
- Get a clear explanation of what’s covered.
- Connect to a human agent if more details are needed.
E-Commerce Returns and Refunds
A customer wants to return an item. Instead of navigating a confusing website, they:
- Chat with a customer service AI that verifies eligibility.
- Get a return label instantly.
- Have the option to schedule a pickup.
Best Practices for Deploying AI Chatbots
How do you get the best performance from AI customer service solutions? Let’s dive in.
1. Use AI and Human Support Together
AI can handle a lot, but it’s not perfect. The best strategy is to:
- Let chatbots manage common issues and gather initial details.
- Enable seamless handoffs to human agents when needed.
- Give agents AI-powered recommendations to speed up resolutions.
2. Keep Training and Improving the Model
Customer service AI chatbots aren’t set-and-forget tools. They need continuous updates to:
- Adapt to new customer behaviors.
- Reduce incorrect responses (AI hallucinations).
- Improve accuracy using real-world feedback.
3. Personalize Responses
Do you like receiving robotic, generic answers when you have a complicated issue? Of course not. AI can provide a more personalized responses by:
- Remembering past interactions.
- Predicting your customers’ needs based on behavior.
- Adjusting its responses to match different communication styles.
4. Prioritize Security and Compliance
Chatbots might have to deal with sensitive information, so you must ensure they follow strict security protocols. This starts in the training stage, where you must anonymize the data before feeding it into the model.
5. Track Key Metrics to Improve Performance
Success isn’t just about automation—it’s about results. Businesses should monitor:
- Resolution rate: How many cases AI handles on its own.
- Escalation rate: How often a chatbot needs human intervention.
- Customer satisfaction scores: How users feel about AI interactions.
- Response time: How quickly issues get resolved.
Challenges and Limitations of AI Chatbots
1. Handling Ambiguous Requests
Not every customer is clear about what they need. AI must be trained to ask clarifying questions before jumping to conclusions.
2. Preventing AI Hallucinations
Sometimes, AI generates incorrect or misleading responses. The best way to prevent this is by:
- Using only verified data sources.
- Setting confidence thresholds for AI-generated answers.
3. Maintaining Trust and Transparency
Customers should always know when they’re talking to AI. It’s important to:
- Clearly disclose AI interactions.
- Offer easy access to human support.
Conclusion
AI customer service solutions have gone beyond simply answering frequently asked questions. Thanks to advances in NLP, machine learning, and backend integrations, chatbots are more efficient than ever. They’re getting closer to handling service integrations as humans would.
Can it work on its own? Not quite yet, support desks still need human ingenuity. The companies that excel in this area will use a clever mix of AI chatbots for customers and AI agents to support service consultants.